Artificial Intelligence in Insurance
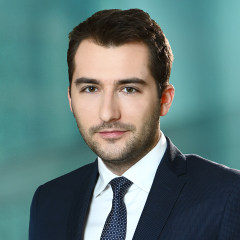

Underwriting, pricing, customer service and actuarial departments have been always using data to manage the risks, create new products, process claims or improve sales and customer experience. Data plays a central role in insurance sector and it is obvious that the importance of data-driven solutions would increase even more in the near future. According to the report, globally stored information grew at 23% per year and insurance sector is not an exception. Recently, there has been an explosion of sources and amount of data stored by insurance companies including:
- policy data,
- market and cash-flow data,
- telematics,
- online and social media activity.
It is increasingly important for insurers to invest in predictive analytics and machine learning solutions to boost the performance of their models and utilize the information stored in their data. This is required to stay competitive in the market, enhance customer satisfaction and meet the regulator requirements.
Key factors for success
Recent survey claims that 40% of "AI startups" in Europe don’t actually use AI. Moreover, there may be a lot of hype about AI in web articles, press and conferences. So, what is the right picture about AI in insurance companies? Undoubtedly, the AI plays increasingly important role in insurance sector. Here are some basic facts:
- the quality and amount of data is constantly increasing (Internet of Things (IoT) sensors for automotive insurance and lifestyle apps for health insurance)
- competitiveness when harness the power of data. An April 2017 Accenture survey found that 79% of insurance executives believe that: “…AI will revolutionize the way insurers gain information from and interact with their customers.”
- number of successful AI startups (StateFarm, Progressive, DataRobot)
However, there exist key factors that management should take into account when planning an AI project:
- Quality and amount of data - data scientists spend 80% of their time for data cleaning and data related tasks. After all it might turn out that the data was too limited to draw meaningful conclusions. Therefore, it is suggested to start a project with a pilot with an aim to assess the data quality first, before going into deep water.
- Qualified data scientists - data scientists in not a programmer, nor actuary or statistician. It is a person that do both, that is why there is such a shortage on the market for true data scientists. An experienced data science team would be able to develop machine learning algorithms, statistically analyze the outputs of a model and decide whether the solution provide a benefit for stakeholders.
- Management with understanding - It is crucial for management to understand basic AI topics and recognize how to utilize the possibilities of data.
Examples
Underwriting and pricing
Today
The underwriting and pricing using old-fashioned models with a lot of manual tasks, expert judgement and tons of paperwork.
Tomorrow
Machine learning algorithms simplify and automate underwriter’s work. Moreover, NLP (Natural Language Processing) methods can be used to analyze application text and automatically draw conclusions. When it comes to the pricing, the conventional methodologies such as burning cost can be greatly improved with stochastic and AI approach to reduce costs and boost predictability. Utilizing the personalized data from Internet of Things (IoT) sensors will allow safer drivers to pay less for auto insurance and people with healthier lifestyles to pay less for health and life insurance.
Claims and loss prediction
Today
Predictions are based on Excel spreadsheets with simple methodologies or econometric models.
Tomorrow
It is extremely important to assess risk and correctly predict claim frequency and loss severity in both life and non-life insurance. Non-linear machine learning models such as random forest or neural networks are able to detect risks early leading to a huge competitive advantage for an insurer. Moreover, automated fraud detection models can prevent losses from fraudulent claims.
Actuarial modeling
Today
Although actuaries use very complex statistical models, they spent a lot of time to change and run them. This corresponds to huge employments costs or high cloud computing expenses.
Tomorrow
Complex actuarial models and processes can be automated in an intelligent way with machine learning models. For instance proxy modelling, currently solved by linear models can be simplified and improved by random forests and neural networks algorithms.
Process Optimization
Today
Claim settlement, actuarial reporting, development of new products and online sales takes departments significant amount of time to plan, develop and use.
Tomorrow
Repetitive and manual elements of these processes should be automated, so that the time saved can be allocated in more interesting tasks. As an example Lemonade’s AI solution is settling a claim in less than three seconds, which is a thousands time faster comparing to traditional methods used in claims departments. Moreover, some actuarial processes build during Solvency II are prone to errors and takes weeks to produce important reports. Why not to simplify the work by reorganizing and optimizing processes in Python, R or MATLAB?