EDA For Pricing Actuaries


Brief definition
Exploratory Data Analysis (EDA) is an important step in any pricing process that involves deep analyses of a dataset in order to better understand its characteristics and dependencies. It is a crucial step for actuaries and pricing analysts in gaining insights into the data and identifying trends and patterns that can improve, enrich and accelerate any further portfolio analysis or risk modelling.
[To accelerate the workflow, Quantee Users are served with a dedicated EDA dashboard template.]
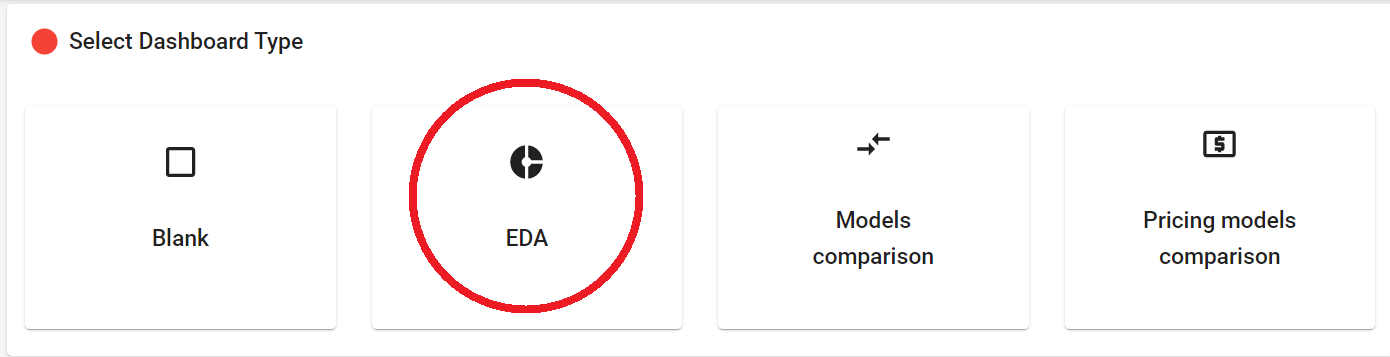
Key aspects
Visual examination
EDA typically begins with a visual examination of the policies, policyholders and claims data, column after column, using various charts and plots to get a sense of the distribution within the data. Such visualisations can help to identify patterns and trends.
[Quantee supports all the most commonly used charts for EDA, and many more. Every graph is fully customisable and interactive.]

Statistic measures and distribution fit
In addition to visualising the data, EDA also involves calculating statistical measures such as mean, quantiles, and standard deviation. These measures can help to identify the distribution and features’ characteristics. The mean and median can give an information about the central tendency, while the standard deviation can provide an indication of the dispersion of the data.
Before modelling it is especially recommended to check, whether the target variable (pure premium/claim frequency/claim severity) fits the assumed distribution. For pure premium models we expect Tweedie family distribution with power between 1 and 2, for claim frequency – Poisson or Negative Binomial distribution, and for claim severity (average claim amount) – Gamma distribution.
[Quantee Users can easily check the distribution fit of a feature. A proper test value (Chi-squared for discrete cases and Kolmogorov-Smirnov for continuous) is displayed above the chart along with the corresponding p-value. Users can select Normal, Poisson, Gamma, Negative Binomial or Inverse Gaussian distribution, and determine whether they want to see the probability density function (PDF) or the cumulative distribution function (CDF).]
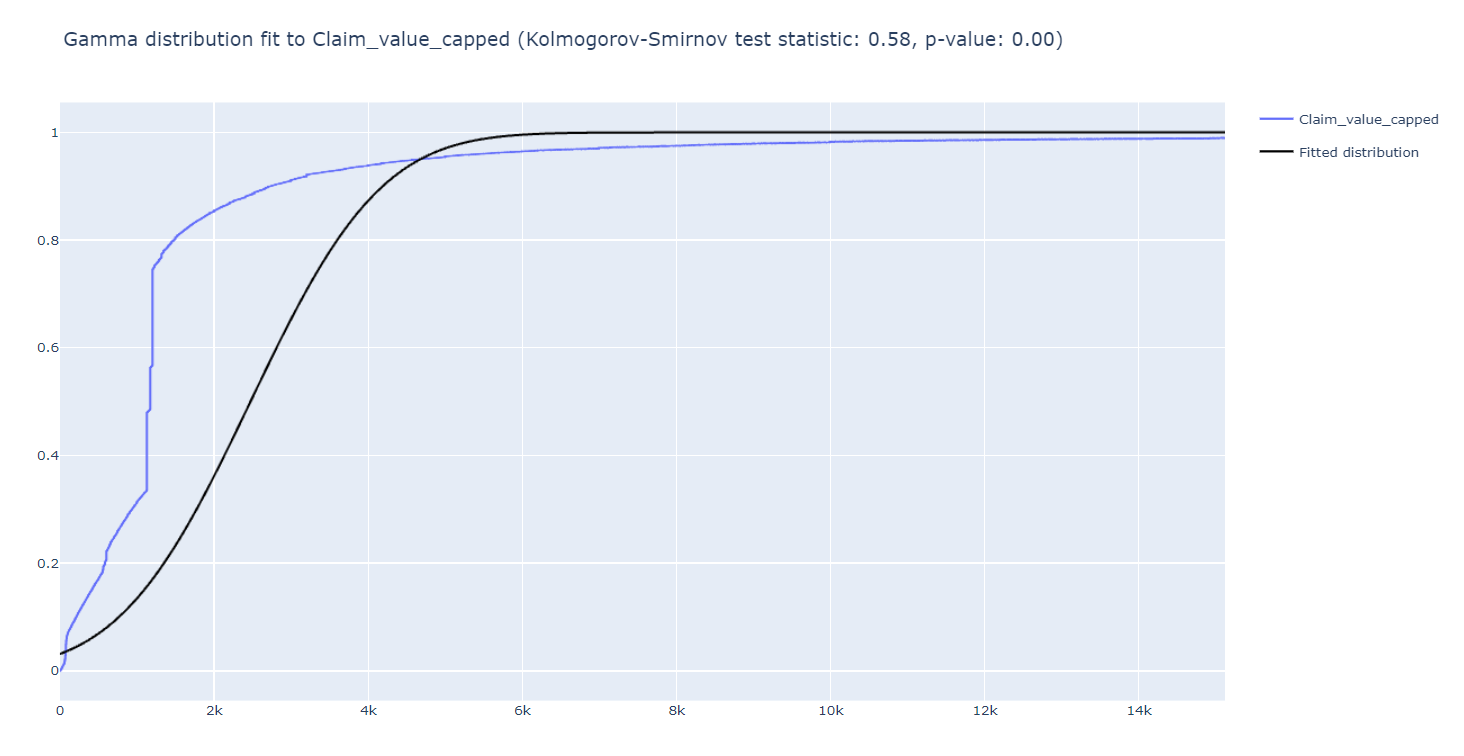
Missing values and outliers
Another key aspect of EDA is to identify and address any potential issues with the data such as missing values (blanks) and outliers.
Blanks can be a particular problem, as they can bias the results of any analysis or modelling that is performed on the data. Sometimes, especially when the dataset was obtained from different systems, there is a high risk of having missing values in policyholder or policy data. Handling them properly may be particularly challenging.
There are numerous strategies that can be applied to take care of missing values, including imputing them with the mean, median or the most frequent value of the column, or simply dropping the rows with blanks (if the dataset won’t be significantly reduced then). What’s crucial, there shouldn’t be any missing values in columns meant to be used as targets or weights in further modelling.
[Quantee platform provides the full flexibility to choose the right strategy to its Users. According to their needs, Users can handle missing values either during data processing (a one-time pre-modelling action) or in the modelling step (with fully deployable imputers). Usually, it’s good to investigate datasets column after column and apply different methods with respect to the number of blanks in a feature, their potential sources and meaning.]

Outliers can also be a concern, as they can have a distortive impact on the results of any further work. It’s especially important for columns like ‘claims amount’, which are prone to have few extremely large values which will affect severity or pure premium models, penalising policyholders’ segment that unluckily happened to have such high claims. Outliers are also important to identify e.g. in policyholder’s age or vehicle/property value.
Numerous techniques can be applied to identify such irregular values, including using statistical measures such as the interquartile range or percentiles, or using visualisations such as box plots or scatter plots. Handling outliers may include removing such observations from the dataset or (preferable) clipping them. In an insurance pricing process actuaries usually cap large claims to a certain level (either fixed or percentile-based).
[Quantee Users are equipped with several tools to take care of outliers – to both detect and mitigate them, including Isolation Forest or Tukey’s algorithm.]
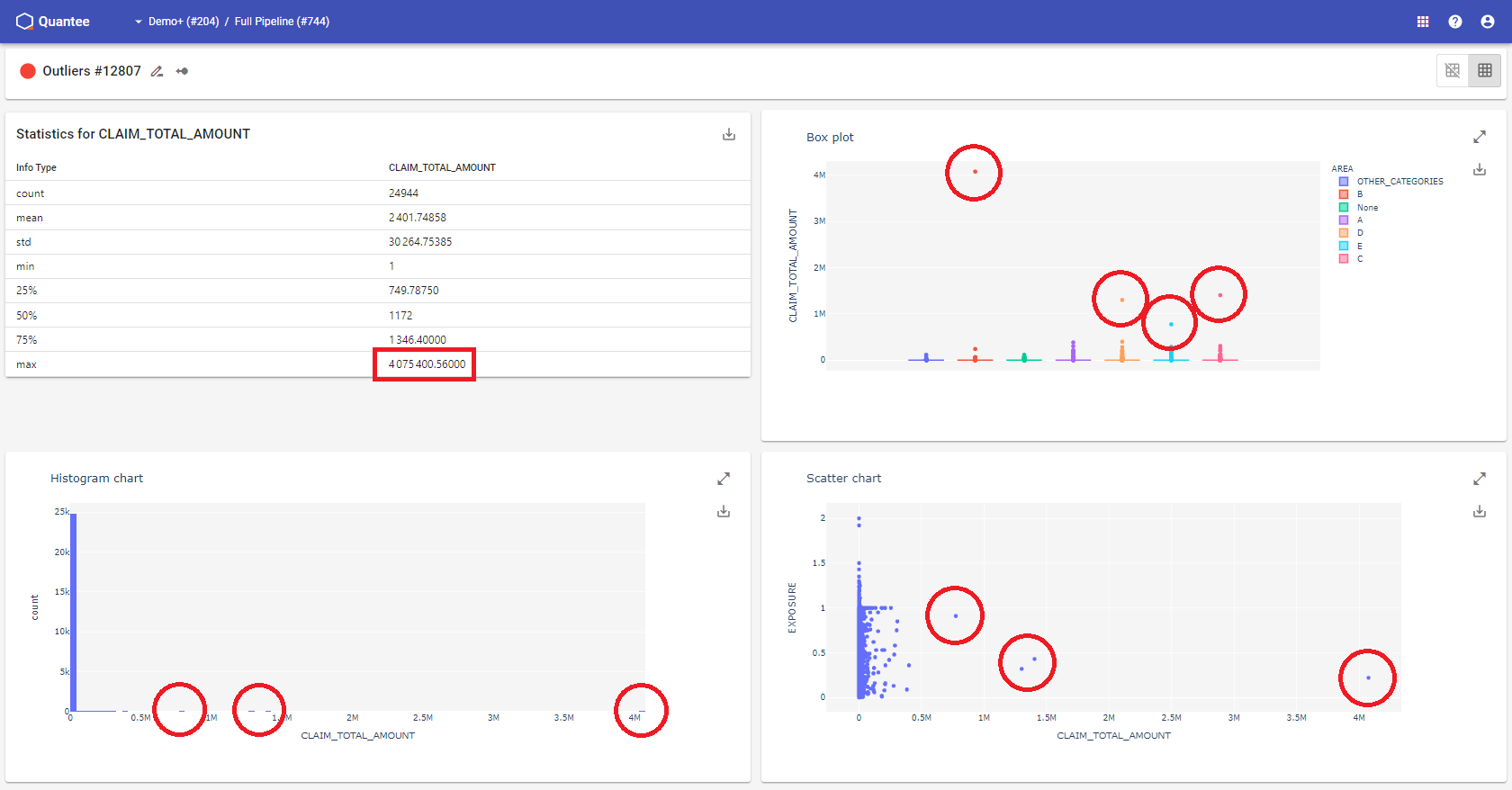
Dependencies
Next important aspect of EDA is understanding the dependencies between different variables in the data. This can be done using techniques such as correlation analysis, which measures the strength and direction of the relationship between two variables. Correlation analysis can help to identify variables that are likely to be important predictors in any further analysis or modelling, and can also help to identify potential multicollinearity issues, where two or more variables are highly correlated with each other, such as usually a policyholder’s age and the risk class (bonus-malus).
[Quantee Users can choose from Pearson, Spearman and Kendall correlations for numeric variables, Cramer’s V for categorical, and p-values of one-way ANOVA for mixed types.]
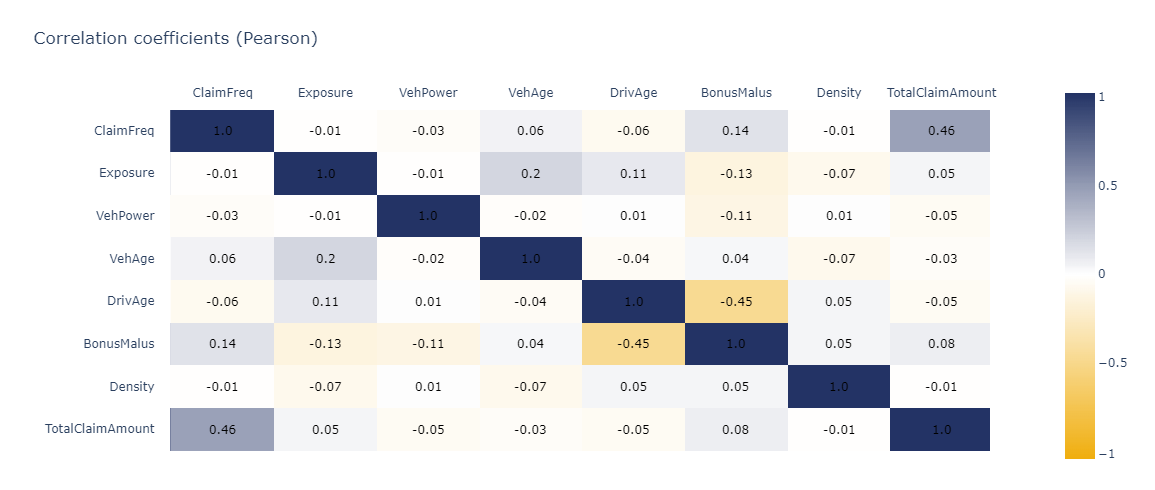
Another technique to look for dependencies in the dataset is using charts with segmentation that can be used to unveil interesting interactions between two dependent variables (which can be later directly applied in the modelling step to increase the predictive power of an estimator).
[Quantee enables segmentation in every suitable type of charts.]
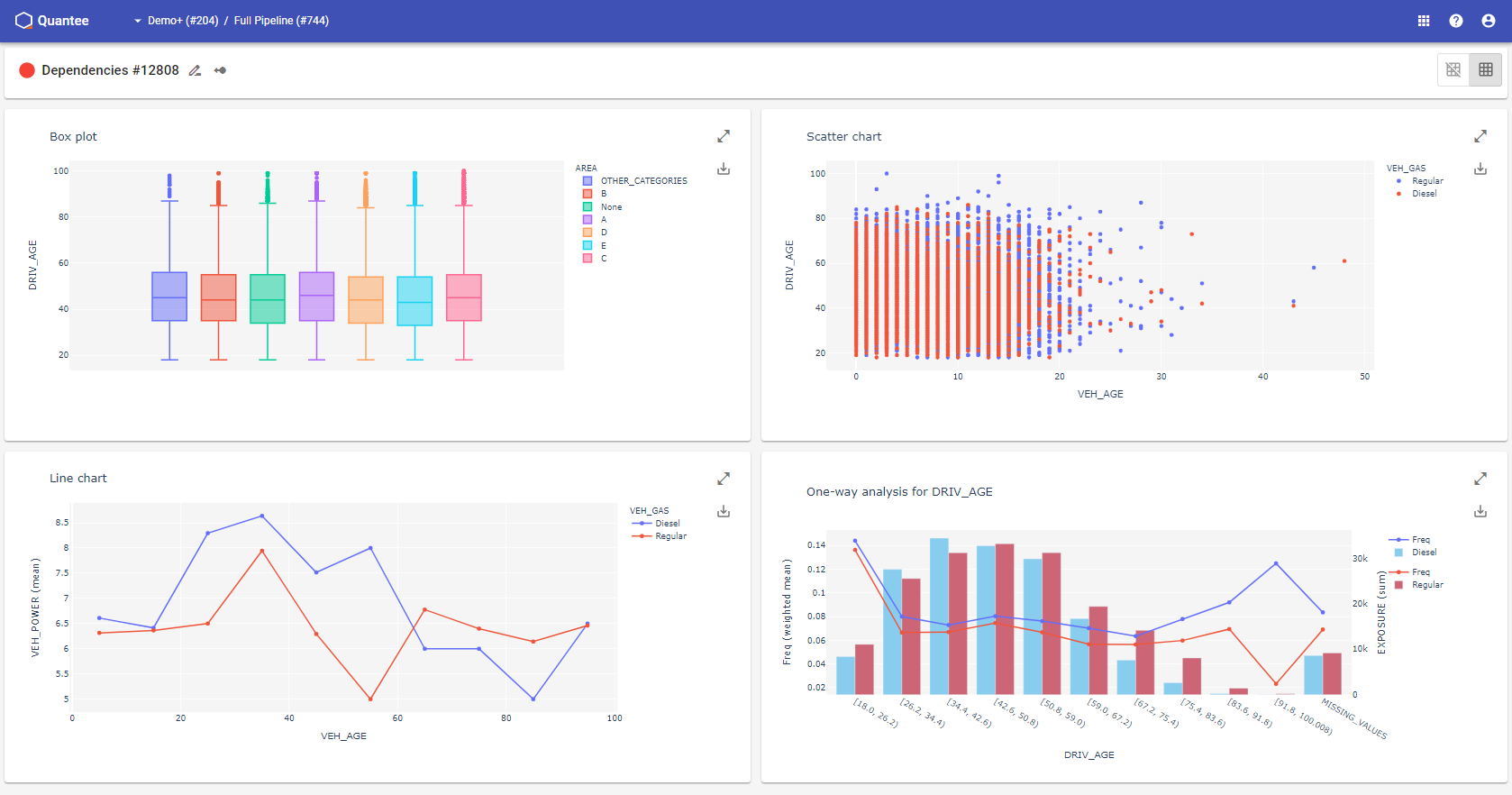
EDA can also involve regression analysis, which helps to understand the relationship between a dependent variable and one or more independent variables. It also helps to identify the strength and direction of such relationships.
[Quantee Users are able to run quick automatic GBM modelling to see Feature Importances for a selected case.]
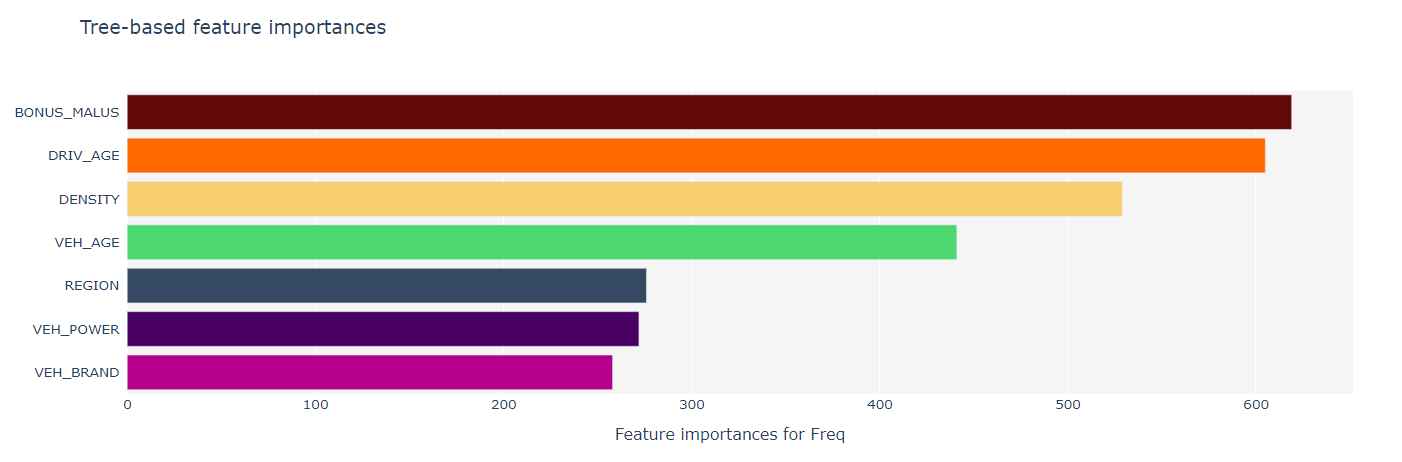
Final remarks
A proper EDA is a long and complex process but certainly worth the time spent. A suitable pricing software helps supercharge and automate this step. What’s more, it’s necessary to keep an open mind and be willing to revisit and modify the analysis as new insights are gained, so flexibility is a must. It is also important to communicate the results of EDA effectively, using visualisations and clear explanations to convey the key findings. By following best practices and using appropriate tools and techniques, EDA can be an invaluable process for both actuaries and pricing leads.

Are you interested in an end-to-end pricing software capable of performing EDA? Contact us and prepare your dashboards in the same place where you process your data, create and maintain your models, and push your well-tailored prices to all sales channels!