Live Monitoring of Demand: A Superior Alternative to Traditional Regression Models for Actuaries
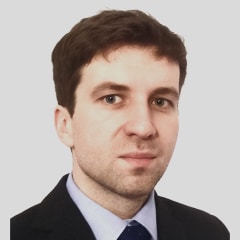
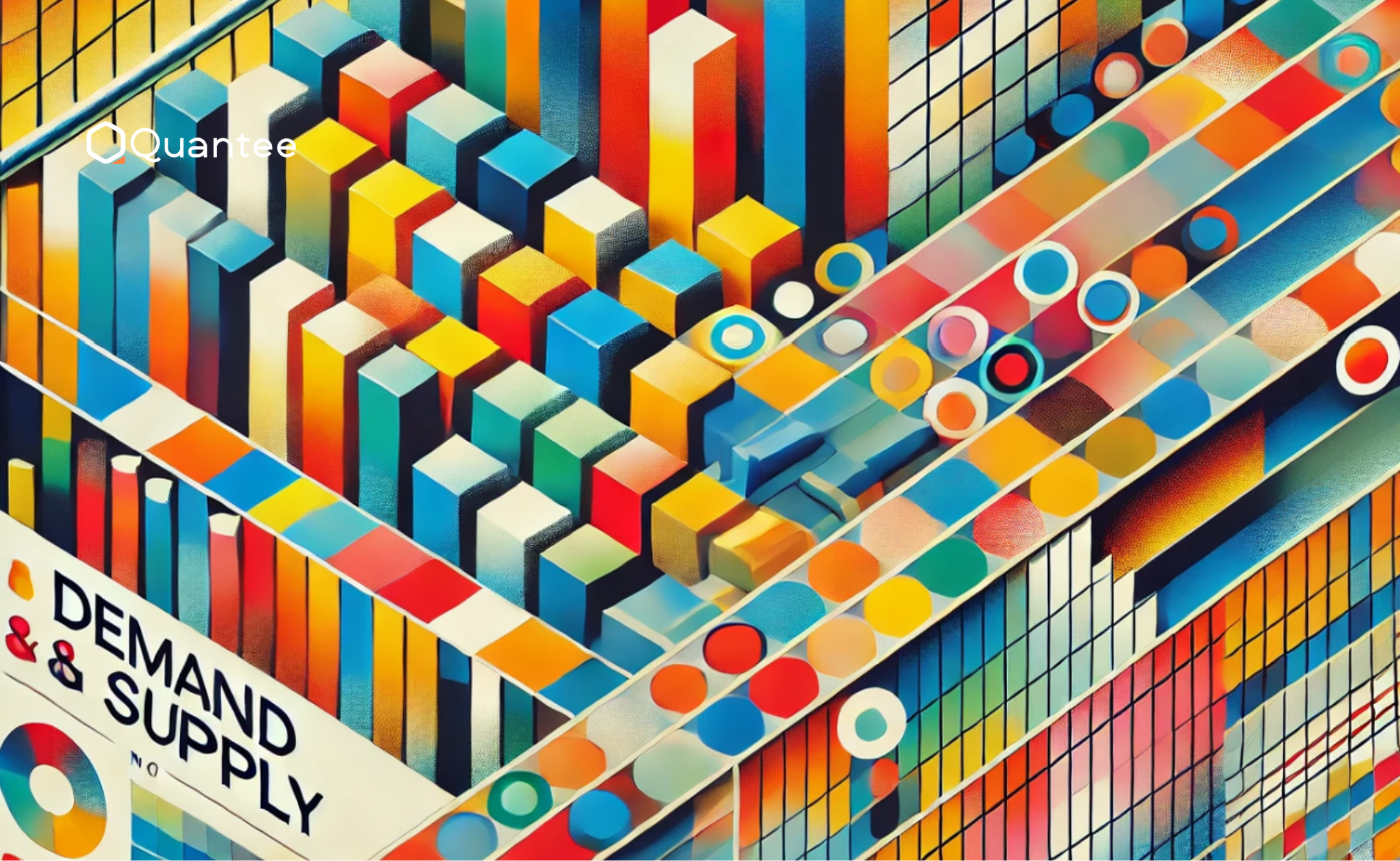
If you’re a hammer, everything looks like a nail.
If you’re an actuary, everything looks like a regression model use case.
It’s the trustiest tool in actuaries’ arsenal: collect some historical data points, pick a function that exists in the same space as those points, pick a method of measuring distance between them, manipulate function parameters to minimise that distance and use the result as a predictor for future of the same phenomenon that points represented, repeat periodically. It’s a well-established tool, perfected over generations of mathematicians, verified in countless use cases in business, in particular by actuaries for risk modelling.
Unsurprisingly, when it comes to modelling demand, regression also becomes a method of choice. Nothing new to it, get points, pick line, fit, done. When browsing through actuarial materials, the most commonly mentioned method for demand modelling appears to be a generalized linear model with logit link function. I’m going to argue that it’s not the best tool for the job, GLM/GAM are far less appropriate for demand than for risk modelling, but there is a better alternative available.
Why is traditional, periodically performed regression not as good for modelling demand as it is for risk, and what to use instead?
The main differences between risk and demand modelling that should influence a choice of methods are:
Stability of behaviour over time
Risk of a certain type of accident happening to a certain type of policyholder does not change easily or often. Probability of purchase of certain product at a certain price by a certain customer may change often, rapidly and without warning when a cheaper or better alternative becomes or ceases to be available. This may mean that a regression model based on last years’ data becomes entirely wrong in a changed market next year.
As observed in data1 “estimating price elasticity shows that more than price, ranking drives demand” – ranking, that is a place amongst other similar products ordered by price, which might change often and fast.
Availability of datapoints
The main driver of purchase probability is price, so to model it well, a broad set of datapoints representing the same type of customers buying (or not) a product at varying prices is needed. This is not often available, as price is a function of customer characteristics, which gives us one data point. Randomised price testing (that is offering a price increased or decreased by a certain percentage to randomly selected subset of customers) may give us more points, still representing only a small part of the whole curve. This may mean that a model is inappropriate for a price outside of tested values.

Time scales
Collecting data points for risk modelling is complex and takes a lot of time. At least the full duration of policy (and possibly consequences of events under cover) for each data point. Demand data point represents a quotation and customer’s decision to accept it or not, which happens in most cases almost instantly. This means that instead of modelling on historical data, demand can be measured almost in real time - by simply splitting customers into groups with similar profiles (and offered price) and counting how many accept and decline their offer over some time span, which may be much less than duration of policy. With small random price variation, price elasticity (that is steepness of demand as a function of price – see our earlier blog post on demand modelling2) can be locally measured.
This solves two problems above – changes in time are less of an issue when measurement is fast. There’s less of a need for a wide set of datapoints covering wide range of possible values, and fitting a whole curve, when objective can simply be measured where needed, which is around price offered or tested as a potential offer. Changes in demand due to behaviour of competition can be detected and acted upon rapidly.
How can this be used?
Most importantly as accurate information about portfolio performance and market changes that influence it. In conjunction with price optimisation algorithm it can be used to adapt to changing market and maximise selected measures, possibly even as a fully automatic algorithm with iterative price adjustments, measurement of impact and search for a price value matching selected goals or constraints. All that without the need to build complex demand models, just by measuring it live at current price points and with small random test movements to detect elasticity. More conservatively, real time measurement can be used to quickly identify discrepancies between traditional demand model used for tariff production and reality (that can be caused by poor model or changes in market) and trigger further investigation or adjustments by actuaries. It can also indirectly provide valuable knowledge about pricing strategy of competition which influences demand, may perhaps even allow to spot errors in risk modelling (unusually high or low demand at risk-derived price may mean that an error in risk estimation has been made).
That being said, live measurement of demand, being not a common and well-established operation in insurance business, comes with its own set of challenges and unknowns, both practical and regulatory. However, it has been employed and even accepted as standard in other businesses, the best example being Uber dynamic pricing3 (which differs from insurance in constraints though, that is the number of available cabs). Perhaps it might in the future become also a more common practice in the insurance world. For more information – get in touch!
References
- https://www.theactuary.com/2022/11/03/model-behaviour-unlocking-potential-price-elasticity-general-insurance
- https://www.quantee.ai/resources/beyond-numbers-unlocking-customer-insights-with-demand-models
- https://www.npr.org/2016/05/17/478266839/this-is-your-brain-on-uber
%2520(1).png)