The quest for the Perfect Price – price tests in insurance pricing
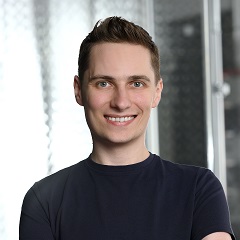
.jpg)
Setting a price
How does one set a price? It’s a non-trivial question, although it might seem otherwise at the beginning. In general, there’s no objective way to calculate or derive a “proper” price of a good or a service. There are multiple factors affecting the amount at which we are willing to sell, like directly attributable costs of creating the product or providing the service, or the expected profit margin. On the other hand, there are multiple factors affecting the consumer’s choice whether to buy or not to, like their needs, or the perceived value relative to the price. So, if the customer needs the product, they are willing to buy for as long they value their money less than the good or service needed. It’s not really an on-point zero-sum game. The “proper” price for a given customer falls in a range of values at the intersection of two ranges: “at least x” from the provider side and “at most y” from the customer side. It may then evolve in time due to supply and demand changes, but it’s a different story. When we understand that the values of price closer to the right end of the price range are less comfortable for the customer, the question arises: “how do we measure the likelihood of them buying the product or service?”. This in turn leads directly to the topics of price elasticity and price optimisation. But before we get to the modelling part, there is data to be collected.
Defining the need
In the context of insurance pricing the premium is a price for a service of risk transfer (which we usually call a product). In order to measure the customer’s price elasticity, we need to gather appropriate data. The usual information we get is the premium at which the policy was sold, and the general risk data. To optimize our price, we need to know how likely it is to sell the policy at various premium levels. Usually, the price is given by the same model for all risk profiles, and so we might be tempted to decrease or increase the whole price structure and see what happens. Unfortunately, this would not work due to several reasons. There’s a need for a rigorous framework which would allow us to test different price levels and draw conclusions from it. Fortunately for us it already exists – it’s called A/B testing.
The A/B (or A/B/X in a more complex case) test originates from XIX century. Its first documented use is in the field of medicine. Next century it found its way in the marketing just to become the top statistical method in e-commerce in 2000s. Today, Netflix, Facebook, and Booking.com run thousands of tests each day. Yes, thousands per day. They want to discover all of the variations that give the most of revenue. What kind of tests are we talking about? Well, it depends.
- Microsoft did tests on how to show promoted links in their search results – this ended up with increasing their ad revenue by 12%!i
- Booking.com daily tests different variations of design of their UI to find out e.g., which size, placement and colour parameters of buttons and fields translate to highest revenue increase.ii
- Netflix tested a “top 10” feature for users hoping it would increase the user engagement. It not only was a success, but its strength seems to increase over time.iii
These companies already know that the “experiment with everything” approach has huge payoffs. So why should not we, the pricing actuaries, develop the same mindset? Having said all that, the question persists: what is the price test? It is a randomised experiment involving at least two variants (A, B, …) of a single variable. It allows to compare customer behaviour when presented with variant A or with variant B. The test involves statistical hypothesis testing to answer the question: Is variant A more effective than variant B? Depending on the context and business need the test might take a different form, but we will stick to the insurance pricing.
Stating the problem
Before we write it down, we need to make an important distinction here. There is also a topic of “scenario testing”, but it’s a completely different thing, which is not the subject of this article. However, it turns out that sometimes people might get confused with the two terms. In principle we talk about the price test whenever we (more or less) randomly assign a price adjustment to every quote or policy. In scenario testing we intend to estimate the overall impact of the change on KPIs and volumes to select for the best outcome. Though the two might seem similar, they are not, as the first (A/B test) assesses the impact in controlled production environment, the second (scenario test) assumes the parameters in the analytical environment. The first one is live, the second is not. The example here could be this:
- Price test: adjust premium by +-2% and observe the impact on the buyer’s decision.
- Scenario test: assume higher share of younger drivers and assess the impact on loss ratio.
Now that we know the difference, let’s go back to the main topic. In the case of price test, the A/B variable is the premium and the behaviour we investigate is buying the policy. From stating the problem this way, we conclude it looks like a classification task where the premium is the main explanatory variable and our target is the flag for conversion or retention (again, depending on the context). So, we need to offer premium at different levels (usually the base level and two small deviations e.g., +/-2%) for the same profile of customers and record whether we got the sale or not. Note that the range of price variation will imply the range at which the optimization can be meaningfully performed. Indeed, if in the experiment we investigate only price levels of 0.99x, 1.00x and 1.01x, then a query for the price level of e.g., 0.96x or 1.05x is out of the scope of the model. The model is always a point of view on the real-world process and aims to capture its core behaviour in the data used to train the model, and then to extrapolate to grey areas. Consider an example where we would like to try and predict a premium for a customer in the future. If the model is trained on data where people are in general aged 18-100, then the model is not suitable to answer this hypothetical question for someone aged 150. It’s just out of the scope.
Understanding the challenges
So, it’s done, case closed? Not really. It turns out there are challenges in gathering the data. It would be best if all policies were sold directly to the clients, but that is not the reality. In real life we see that usually only a part of GWP comes from direct channels where the insurer has full control over what data they gather, and they do that. In remaining cases there are external sales channels which may be less keen to share the data or less skilled to collect them properly. In some cases, the intermediaries might want to sell the demand data at a price which is very high and might not be acceptable by some companies. On the other hand, the agents/brokers might be reluctant to share the data because they are protecting their business and don’t want to risk that the insurer will take over their pool of clients through the direct channel. Even if they are willing to share the data, they might not want to share all of it and give us some skewed data. There might also be the case where they simply don’t keep all of the information and will share only the datapoints they consider “good”. In a situation like this we risk introducing the survivor bias, where we won’t see the cases of customers that did not want to buy and were less likely to be convinced. On the other hand, even if the insurer does have a steady access to relatively large portion of the sales data, they might struggle to integrate and use it.
Imagining the ideal
The data quality is key in data science. If we want to move to the next phase (modelling) we must make sure the data is properly collected. Ideally, we need to know of all cases when our price model was used to provide a premium value for a customer. In other words: to control the only source of price quotes going to all channels. When we commercialize complex price structures involving multiple price rules, we must know which price came from which model component. Especially when at least one of the components is a price variation used to conduct the A/B test.
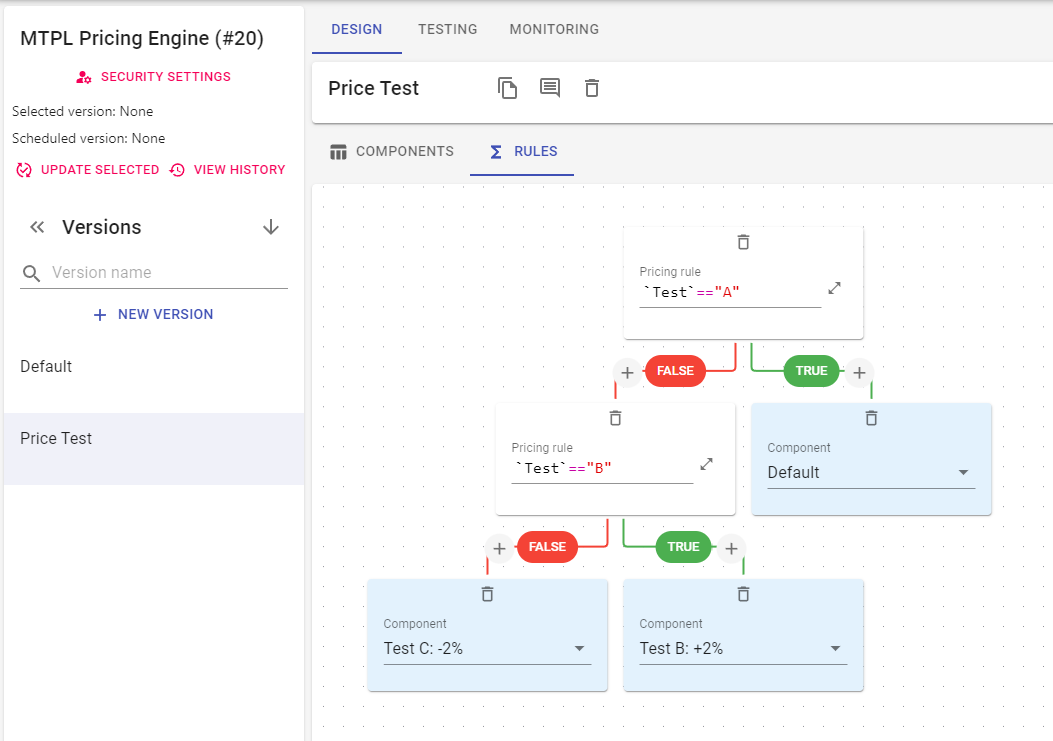
So, the optimal solution would be something like an API endpoint controlled by the insurer. The endpoint leads to a pricing engine which evaluates the price model, sends back the premium value, and saves both the request and the response to it. The engine should be able to evaluate price rules on the data parameters sent to it and based on that reroute the datapoint to either the base model or it’s variation. Note here that ideally the split between variants A and B should be random. However, due to local regulations it might be better to introduce a pseudo-random method (e.g., a rule based on a trait which is more or less evenly spread across whole population, like the day of birth) to enable recreation of the price for the given request. Since both the base model and the variation have their unique identifiers, we would be able to split the data between variants A and B and perform hypothesis testing. Next in line is demand modelling.
Wrapping it up
To summarise the price tests (or A/B tests in general) are randomised live tests as opposed to scenario testing (offline with assumed parameter values). They are means to obtain high quality data which can be used to find out the best possible price for any given customer profile.
Quantee supports pricing actuaries not only in their modelling, but also enables them to perform price tests and gather priceless (although containing the price!) high quality data. Would you like to learn more about our Pricing Engine technology? Give us a call!
%20(1).png)